My first choice for a programming language for solving our graph problem is Python, with its “batteries included” philosophy, and diversity of native data structures. Data structures such as lists, dequeues, and dictionaries seem more of an afterthought for C++ and Java. My past experience with Python is extremely limited, but I am not on deadline, and can use the brain exercise.
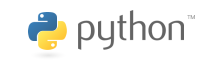
A quick search of graph theory libraries for Python or R turns up NetworkX. The library has built-in solvers for shortest paths, but not necessarily for the longest path we are looking for. But at least I could use their built-in data structures to create my own solver, if necessary.
(In a previous career as a chip designer decades ago, I used awk to solve graph theory problems on huge CMOS netlists because of its built-in associative memory, but let’s stick with a language known to be much more modern and readable, Python.)
An insight about bridges might make our problem easier to solve.
Seven Bridges of Königsberg
Consider these 7 bridges in the ancient city of Königsberg in Prussia. Find a walk through the city that crosses each bridge once and only once.
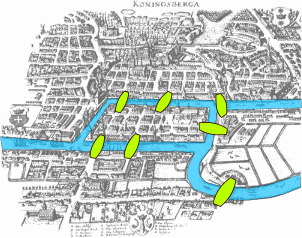
We transform this problem into a graph, where each of 4 land masses is a node, and each bridge is an edge.
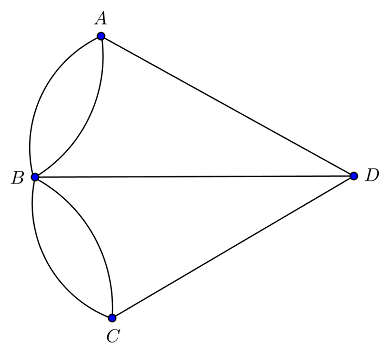
Euler explained that, except for the beginning and end of the walk, whenever you enter a node you must leave the node. So except for the beginning and end, all nodes must have an even number of edges. In this problem, all nodes have an odd number of edges, so a solution is impossible.
Continue reading “Trails and Graph Theory 2: Bridges”